Using AI to improve the analysis of 3D biological images
Scientists have developed a new machine learning model, known as Affinity-VAE, that improves the analysis of 3D biological images in fields such as cryo-electron tomography (cryo-ET).
By making use of prior knowledge about protein structures, the model can identify clusters of similar molecules more accurately than current, manually assisted methods – even in crowded or unclear images. This breakthrough not only enhances researchers’ ability to identify proteins in challenging datasets but also provides information about a molecule’s orientation and shape, which could support further experiments.
Cryo-ET is a powerful, high-resolution imaging technique that has the potential to revolutionise our understanding of molecular and cellular biology. Affinity-VAE addresses the difficulty of extracting useful information from these images, while improved detection of proteins could help advance fields such as drug discovery or disease diagnosis.
The research grew out of a challenge posed by Franklin scientists to members of an Alan Turing Institute Data Study Group: could AI identify patterns of molecules in 3D cryo-ET tissue images without being given any example molecules to target? The question proved so interesting that a follow-up group was formed – composed of researchers from the Franklin, the Turing and the Collaborative Computational Project for Electron cryo-Microscopy (CCP-EM), based in Scientific Computing at the Science and Technology Facilities Council (STFC) – to continue working on a solution.
Dr Mark Basham, Science Director and Challenge Lead at the Franklin, is one of the paper’s senior authors. He says: “This paper represents a unique approach to automatically identifying multiple different proteins in 3D tomographic data. What’s exciting is that it doesn’t just find the proteins we know about – it also helps reveal ones we weren’t aware of. By augmenting our machine learning models using prior scientific knowledge about protein structures, it is able to generalise and make useful predictions even when it encounters proteins it hasn’t seen before, which is a big step forward in the field. It offers an efficient way of analysing complex, noisy data and could help us discover new insights into what’s really happening within cells.”
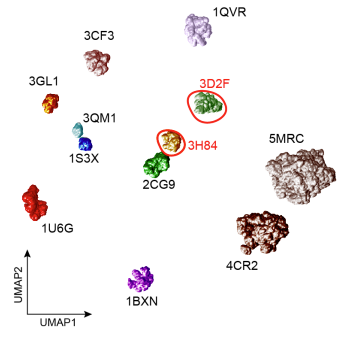
First author Dr Marjan Famili, Research Associate at the Alan Turing Institute, says: “Affinity-VAE was developed to be a truly generative model that can help our understanding of unseen proteins in cryo-ET images based on their similarity to known proteins. Protein similarity is a scientific input for the model which is then reflected in its learned representation. Affinity-VAE benefits from a modular structure with multiple decoders and it can disentangle the object pose from structural similarity as well as disregarding the noise. This leads to an interpretable latent representation from which scientific meaning can be inferred. Affinity-VAE is a versatile model which has been applied to various data types including astrophysical images, transcriptomic data and environmental data.”
Dr Tom Burnley, Leader of the Molecular and Cellular Electron Microscopy Group at STFC Scientific Computing, adds: “This exciting work results from a tremendous collaboration with researchers at the Franklin and the Turing. The tools we developed are now ready to be optimised for use with cryo-ET data collected at the Franklin and other institutes worldwide. If successful, this will allow researchers to maximise the information gathered from in situ experiments, revealing cellular content at close to atomic detail and transforming our understanding of protein and cellular function.”
The study is based on realistic simulated data, and the team’s goals now are to validate the findings experimentally and develop the model to be able to analyse bigger sets of imaging data. Affinity-VAE is open-source software available for researchers anywhere to use via GitHub.
Access the software via GitHub
The paper Affinity-VAE: incorporating prior knowledge in representation learning from scientific images was presented at the European Conference on Computer Vision, and is openly available via ArXiv.